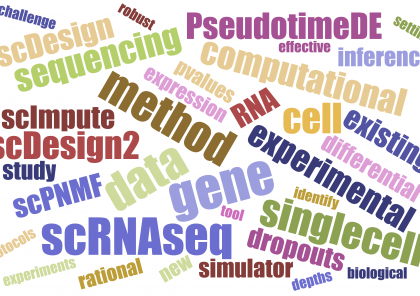
The introduction of single-cell RNA-sequencing (scRNA-seq) over the last seven years has further revolutionized biomedical sciences by revealing the genome-wide gene expression levels within individual cells, in contrast to the traditional RNA-seq, now called the bulk RNA-seq, that only provides average gene expression levels across a batch of cells. The scRNA-seq technologies enabled researchers to identify cell subtypes, to elucidate the key genes that characterize each cell, and to demonstrate that cells are more heterogeneous than previously thought. However, the technologies faced several computational challenges, including how to handle excess zeros, how to decide the number of cells to sequence (“sample size”) and the sequencing depth (“budget”) in experimental design, as well as how to effectively benchmark computational methods for scRNA-seq data analysis.
Previously, we had success in developing a popular imputation method, scImpute [1], to address the excess zero issue in scRNA-seq data. To address the remaining issues, we developed a statistical simulator scDesign [2], which was selected by the ISMB conference (a top conference of bioinformatics) and published in Bioinformatics, for guiding the experimental design and computational method benchmarking in the scRNA-seq field. In our new work [3], selected by the RECOMB conference (another top conference of bioinformatics) and published in Genome Biology, we developed scDesign2 by advancing scDesign and allowing its generative model to include gene correlations, so that its synthetic data can help benchmark “multivariate” computational methods that simultaneously consider many genes as variables.
Powered by scDesign, we performed systematic benchmarking of existing computational methods that detect doublets, i.e., “false cells” that consist of more than one actual cell, from scRNA-seq data. Our benchmarking results [4] were published in Cell Systems.
Moreover, we developed PseudotimeDE, a statistical method that conducts valid inference of differential gene expression along cell pseudotime (i.e., an inferred temporal order of cells) with well-calibrated P-values from scRNA-seq data. We also developed scPNMF, a method built upon the projective non-negative matrix factorization algorithm, for learning sparse gene encoding of single cells; scPNMF enables the selection of a small number of informative genes for designing a more accurate experiment at a lower cost. Both PseudotimeDE and scPNMF are effective tools for extracting hidden information from scRNA-seq data. PseudotimeDE was recently published in Genome Biology [5], and scPNMF was selected by the ISMB conference and published in Bioinformatics [6].
References:
[1] Li, W.V. and Li, J.J. (2018). An accurate and robust imputation method scImpute for single-cell RNA-seq data. Nature Communications 9:997. [ PDF ]
[2] Li, W.V. and Li, J.J. (2019). A statistical simulator scDesign for rational scRNA-seq experimental design. Bioinformatics 35(14):i41–i50. [ PDF ]
[3] Sun, T., Song, D., Li, W.V., and Li, J.J. (2021). scDesign2: a transparent simulator that generates high-fidelity single-cell gene expression count data with gene correlations captured. Genome Biology 22:163. [ PDF ]
[4] Xi, N.M. and Li, J.J. (2021). Benchmarking computational doublet-detection methods for single-cell RNA sequencing data. Cell Systems 12:1-19. [ PDF ]
[5] Song, D. and Li, J.J. (2021). PseudotimeDE: inference of differential gene expression along cell pseudotime with well-calibrated p-values from single-cell RNA sequencing data. Genome Biology 22:124. [ PDF ]
[6] Song, D., Li, K., Hemminger, Z., Wollman, R., and Li, J.J. (2021). scPNMF: sparse gene encoding of single cells to facilitate gene selection for targeted gene profiling. Bioinformatics 37(Supplement_1):i358-i366. [ PDF ]
Associated Researchers: