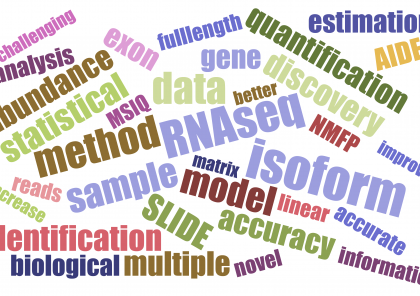
The advancement of next-generation high-throughput sequencing technologies has been revolutionizing genomic studies in the last decade. In particular, the RNA sequencing (RNA-seq) technology, which has deep coverage and base level resolution, enables investigating human and other eukaryotic species’ transcriptomes (i.e., sets of RNA molecules in cells) with unprecedented detail and clarity. Unlike previous technologies such as microarrays, RNA-seq provides information on alternative splicing (i.e., how is the DNA of one gene possibly transcribed into multiple RNA sequences) as well as gene expression on a genome-wide scale without requiring prior knowledge on RNA sequences. This superior advantage of RNA-seq enables the discovery of novel RNA isoforms (i.e., RNA molecules transcribed from the same gene but having different sequences) and the estimation of isoform expression, two crucial steps to understanding molecular mechanisms of diseases and other biological phenomena, in a high-throughput manner. Before the invention of RNA-seq, RNA isoforms have been discovered on a gene-to-gene basis, and the discovered isoforms are recorded inannotations (i.e., databases of genes and their RNA isoforms). Previous methods that aim to discover and quantify RNA isoforms from RNA-Seq data belong to two categories: “annotation-based” and “annotation-free”. The former utilizes existing annotations to aid isoform discovery, but is hindered by the incompleteness of annotations. The latter uses no annotation information but directly assembles RNA isoforms from RNA-Seq data; however, it is affected by widespread non-systematic noise and biases in the data.
In [1], we developed a method SLIDE (Sparse Linear modeling of RNA-seq data for Isoform Discovery and abundance Estimation), which defines a new category—“annotation-aided” methods for discovering and quantifying RNA isoforms from RNA-seq data. SLIDE, which uses a stochastic approach to account for RNA-seq noise and borrows information from known isoform structures, is an annotation-aided method. Combining information from both annotations and RNA-seq data, SLIDE has the advantage of being more capable to find novel isoforms (compared to annotation-based methods) as well as being more robust to data noise and biases (compared to annotation-free methods). After its publication, SLIDE has received considerable attention from the RNA-seq field (cited for 91 times) and has been adapted for use by the modENCODE consortium (a nationwide project that aimed to identify all of the sequence-based functional elements in the genomes of two model organisms, the nematode worm C. elegans and the fruit fly D. melanogaster) (Brown et al, Nature, 2014; Boley et al, Nature Biotechnology, 2014). In an independent assessment of multiple isoform discovery methods conducted by the RGASP consortium, SLIDE achieved top performance as compared to other methods (Steijger et al, Nature Methods, 2013).
However, the isoform discovery task remains a great challenge in studying human transcriptomes, mostly because the complex alternative splicing mechanisms of human genes lead to an ultra large search space of possible RNA isoforms. Therefore, in [2] we developed a method NMFP (Nonnegative Matrix Factorization based Preselection) as an upstream preselection step that significantly improves the precision and recall rates of SLIDE and other methods for isoform discovery. Moreover, to further improve the accuracy of estimating isoform expression, in [3] we developed an umbrella statistical method MSIQ (joint modeling of Multiple RNA-seq Samples for accurate Isoform Quantification) to jointly use the information in multiple RNA-seq replicates while accounting for possible replicate heterogeneity.
In our most recent work [4], which was published as a cover story in Genome Research, we developed a novel statistical method, AIDE (Annotation-aided Isoform Discovery and abundance Estimation), the first approach to directly control false isoform discoveries from RNA-seq data by implementing the statistical model selection principle. Solving the isoform discovery problem in a stepwise and conservative manner, AIDE prioritizes the annotated isoforms and precisely identifies novel isoforms whose addition significantly improves the explanation of RNA-seq data. Compared with existing isoform discovery methods, AIDE has the advantage of achieving the highest precision rate. I was interviewed by Robyn Williams at ABC Australia to introduce our AIDE method at the Science Show he hosted (URL).
In summary, NMFP, MSIQ, and AIDE are tools for isoform/transcript-level analysis on short-read RNA-seq data. We expect them to facilitate the reuse of large-scale short-read RNA-seq data sets in public repositories for new studies. For our review of statistical modeling of RNA-seq data, please see [5].
References:
Associated Researchers: